Data Analysis Paperwork
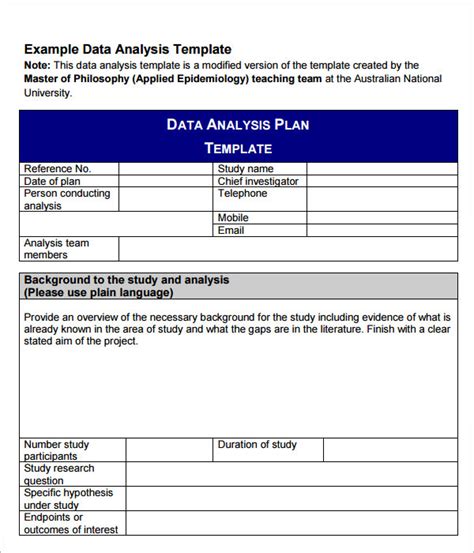
Introduction to Data Analysis Paperwork
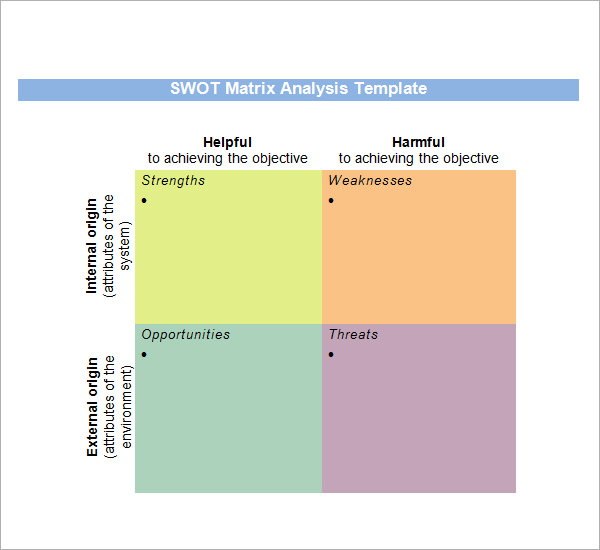
Data analysis paperwork is a crucial aspect of any research or business project, as it involves the process of collecting, organizing, and analyzing data to draw meaningful conclusions. The paperwork involved in data analysis can be daunting, but it is essential to ensure that the data is accurate, reliable, and relevant to the research question or business objective. In this blog post, we will explore the importance of data analysis paperwork, the steps involved in the process, and the tools and techniques used to manage and analyze data.
Importance of Data Analysis Paperwork
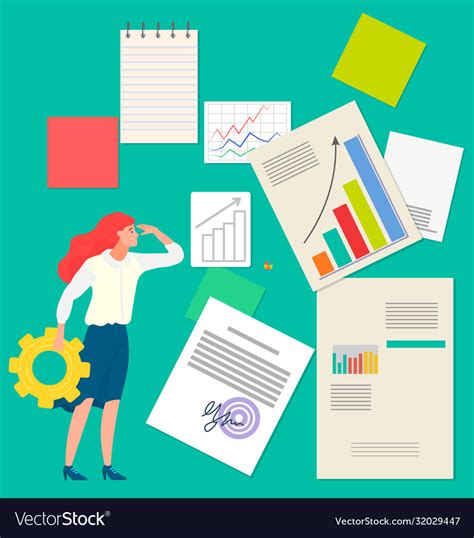
Data analysis paperwork is essential for several reasons. Firstly, it helps to ensure that the data collected is accurate and reliable, which is critical for making informed decisions. Secondly, it enables researchers and businesses to identify patterns and trends in the data, which can inform strategy and decision-making. Finally, data analysis paperwork provides a transparent and reproducible record of the data collection and analysis process, which is essential for validating the findings and ensuring that the results are trustworthy.
Steps Involved in Data Analysis Paperwork
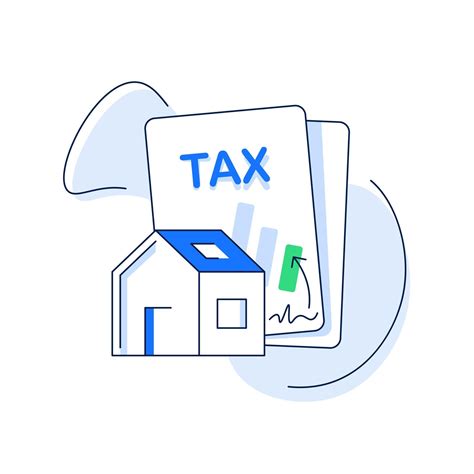
The data analysis paperwork process typically involves the following steps: * Data collection: This involves gathering data from various sources, such as surveys, interviews, or observations. * Data cleaning: This involves checking the data for errors, inconsistencies, and missing values, and cleaning it up to ensure that it is accurate and reliable. * Data organization: This involves organizing the data into a format that is suitable for analysis, such as a spreadsheet or database. * Data analysis: This involves using statistical techniques and tools to analyze the data and identify patterns and trends. * Data interpretation: This involves interpreting the results of the analysis and drawing meaningful conclusions.
Tools and Techniques for Data Analysis Paperwork
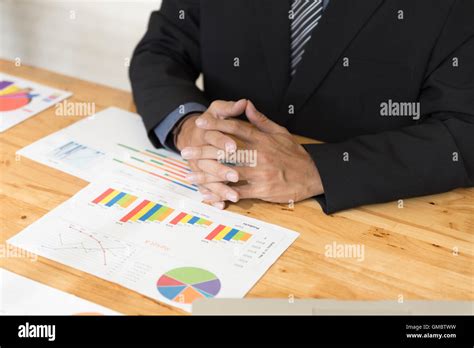
There are several tools and techniques that can be used to manage and analyze data, including: * Spreadsheets: Spreadsheets, such as Microsoft Excel or Google Sheets, are commonly used for data analysis and provide a range of tools and functions for data manipulation and analysis. * Statistical software: Statistical software, such as R or SPSS, provides advanced tools and techniques for data analysis, including regression analysis, hypothesis testing, and data visualization. * Data visualization tools: Data visualization tools, such as Tableau or Power BI, provide a range of tools and techniques for visualizing data, including charts, graphs, and maps. * Machine learning algorithms: Machine learning algorithms, such as decision trees or neural networks, can be used to analyze large datasets and identify patterns and trends.
Tool | Description |
---|---|
Spreadsheets | Used for data analysis and provide a range of tools and functions for data manipulation and analysis |
Statistical software | Provides advanced tools and techniques for data analysis, including regression analysis, hypothesis testing, and data visualization |
Data visualization tools | Provides a range of tools and techniques for visualizing data, including charts, graphs, and maps |
Machine learning algorithms | Can be used to analyze large datasets and identify patterns and trends |
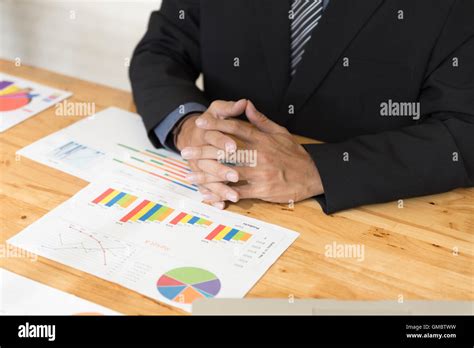
💡 Note: The choice of tool or technique will depend on the specific requirements of the project and the level of complexity involved.
Best Practices for Data Analysis Paperwork
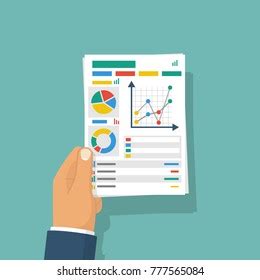
To ensure that data analysis paperwork is effective and efficient, it is essential to follow best practices, including: * Keeping a record of the data collection and analysis process: This provides a transparent and reproducible record of the data collection and analysis process, which is essential for validating the findings and ensuring that the results are trustworthy. * Using version control: This involves using tools, such as Git or SVN, to track changes to the data and analysis code, which helps to ensure that the data and analysis are reproducible and trustworthy. * Documenting the analysis: This involves documenting the analysis, including the methods used, the results obtained, and the conclusions drawn, which provides a clear and transparent record of the analysis.
Common Challenges in Data Analysis Paperwork
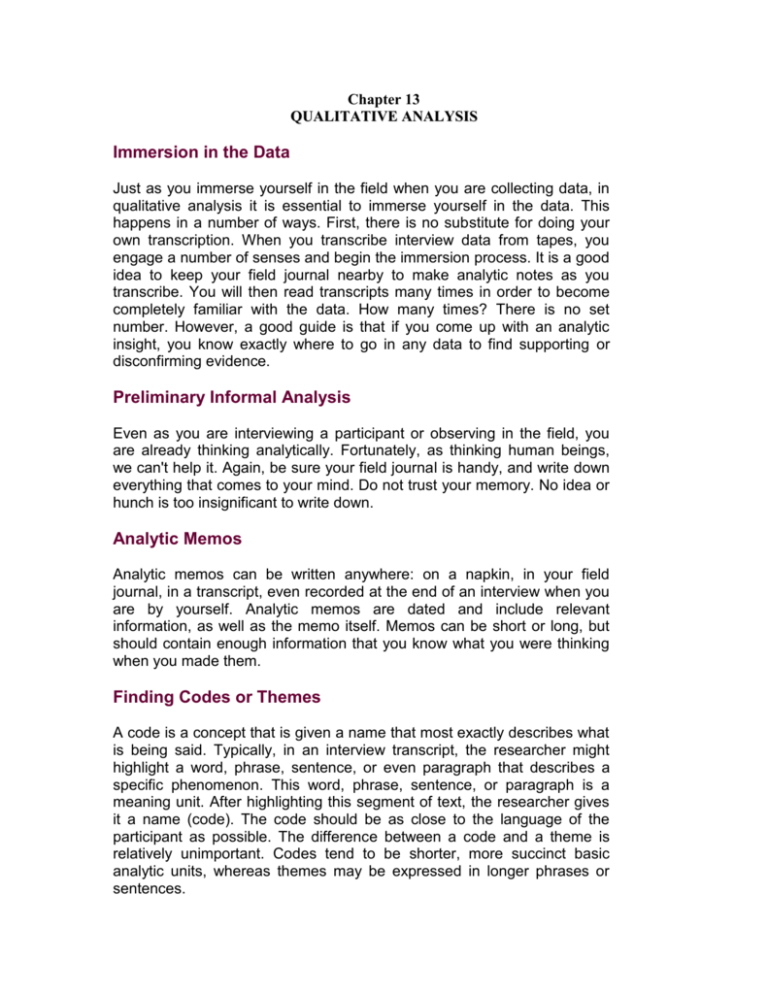
Data analysis paperwork can be challenging, and common challenges include: * Data quality issues: Data quality issues, such as missing or inconsistent data, can make it difficult to analyze the data and draw meaningful conclusions. * Complexity of the analysis: The analysis can be complex, especially when dealing with large datasets or complex statistical techniques. * Time and resource constraints: Data analysis paperwork can be time-consuming and resource-intensive, which can make it challenging to complete the analysis within the required timeframe.
📝 Note: To overcome these challenges, it is essential to have a clear understanding of the research question or business objective, to use the right tools and techniques, and to follow best practices.
To summarize the key points, data analysis paperwork is a crucial aspect of any research or business project, and it involves the process of collecting, organizing, and analyzing data to draw meaningful conclusions. The importance of data analysis paperwork lies in its ability to ensure that the data is accurate, reliable, and relevant to the research question or business objective. By following best practices, using the right tools and techniques, and being aware of common challenges, data analysis paperwork can be effective and efficient.
What is data analysis paperwork?
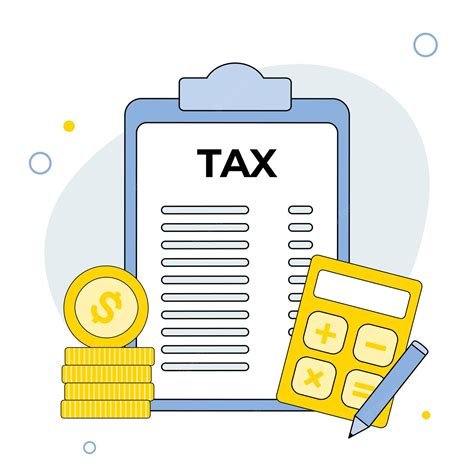
+
Data analysis paperwork is the process of collecting, organizing, and analyzing data to draw meaningful conclusions.
Why is data analysis paperwork important?
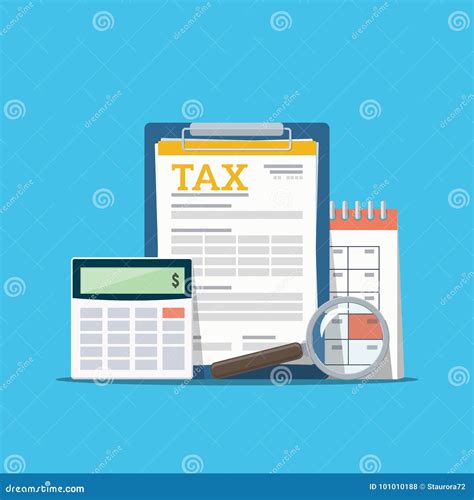
+
Data analysis paperwork is important because it ensures that the data is accurate, reliable, and relevant to the research question or business objective.
What are the steps involved in data analysis paperwork?
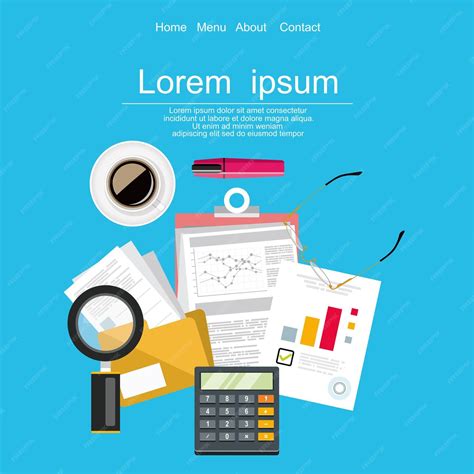
+
The steps involved in data analysis paperwork include data collection, data cleaning, data organization, data analysis, and data interpretation.